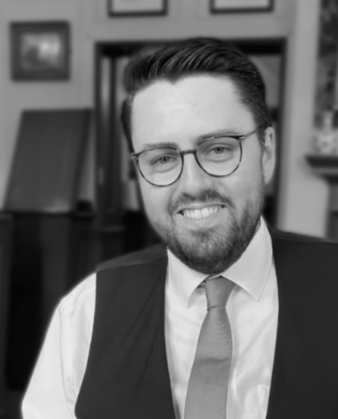
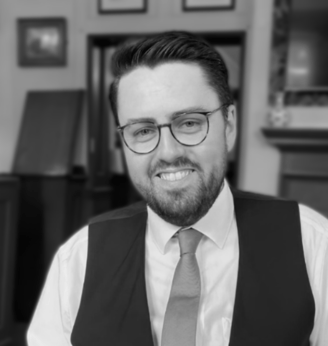
I'm a postdoctoral researcher working in astrophysics.
Currently I use machine learning techniques to better understand how gravitational lensing can tell us about dark matter.
In general, I'm interested in any application of statistics and computation to interesting problems, especially if it involves machine learning, Bayesian inference, or high-performance computing.
CURRENTLY
Astrophysics Group
Imperial College London
UK
PREVIOUSLY
Talks
UPCOMING
Debating the potential of machine learning in astronomical surveys 2
IAP Paris, France / Flatiron Institute, New York, USA | Nov/Dev 2023 | link
PAST
IAU Symposium 381: strong gravitational lensing in the era of big data
Otranto, Italy | June 2023 | link
Nature of dark matter on small scales
Yale seminar series, over zoom | Sep 2023
SHARP collaboration meeting
Piemonte, Italy | June 2023
Origins data science workshop
Origins Excellence Cluster, Garching, Germany | Jan 2023 | link
Munich dark matter meeting
MPA, Garching, Germany | Dec 2022 | link
Euclid strong lens science working group meeting
Marseille, over Zoom | Dec 2022
Debating the potential of machine learning in astronomical surveys
IAP Paris, France | Oct 2021 | link | video below
Projects
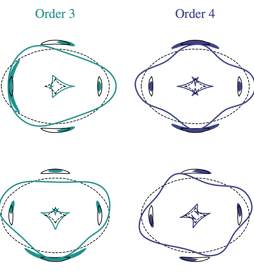
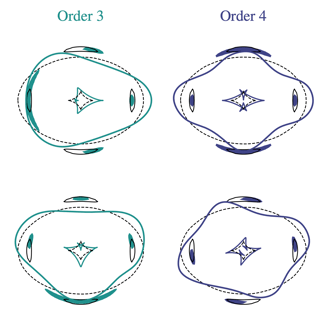
Using our machine learning method developed previously, we examined the effect of angular complexity in the lens model on dark matter substructure detection. Using CNNs trained on data with and without angular multipole perturbations, we found that small angular perturbations in the lens can cause a significant rate of false positive substructure detections.
ANGULAR COMPLEXITY IN STRONG LENS SUBSTRUCTURE DETECTION
FAST SENSITIVITY MAPPING AND EUCLID FORECASTS
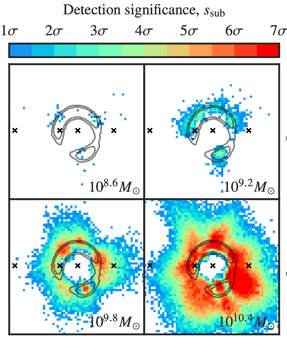
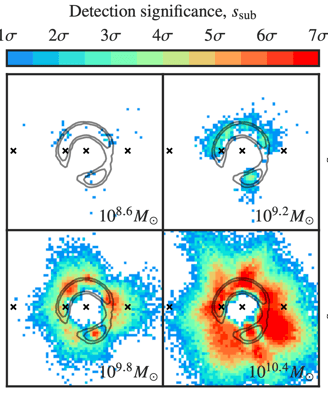
When strong lensing is used to detect dark matter subhaloes, it is essential to know the sensitivity of the data, that is, what's the smallest DM subhalo that one could detect, if it were there. This information turns a set of subhalo detections and non-detections into an inference on the true dark matter model of the universe.
We developed a machine learning method to map the sensitivity of strong lens observations. This was previously a very expensive and time consuming part of the analysis. With fast and cheap sensitivity mapping, we can learn much more about the way that dark matter subhaloes effect strong gravitational lenses, allowing us to better focus our detection efforts.
Using our new method, we computed sensitivity maps for 16k simulated Euclid strong lens images, allowing us to forecast the number of detections we expect in that survey.
GALAXY MASS PROFILES FROM STRONG GRAVITATIONAL LENSING